1Universitätsklinikum Heidelberg Klinik für Innere Med. III, Kardiologie, Angiologie u. Pneumologie Heidelberg, Deutschland
Background: The intersection of molecular biology, cardiology and machine learning (ML) offers a novel and promising approach to validate the role of microRNAs (miRNA) in cardiovascular disease (CVD). In this work, we systematically explored and evaluated the diagnostic potential of miRNA signatures using ML approaches for a range of CVD, including ACS, CAD, DCM, and HFrEF in a multicentric, prospective cohort with a particular focus on validating miRNAs that have been previously identified in the literature. We also aimed to investigate the diagnostic impact on patient survival and disease severity.
Methods: We assessed genome-wide miRNA expression profiles in a total of 1,209 cardiovascular patients and 849 controls, all participants of the multicentric BestAgeing study. A comprehensive database literature search was performed using text mining tools (miRetrieve and the OpenAI gpt-3.5-turbo model) to identify original studies focusing on the role of miRNAs in CVD and their associated diagnostic biomarkers. The top hits were extracted and used for validation in both a differential expression analysis and multivariate ML signatures. We trained disease-specific binary classification models using repeated 5-fold cross-validation and subsequently evaluated the refined models on a blinded 25% test set. We then evaluated the influence of the diagnostic model probabilities (divided into low, mid, and high tertiles of diagnostic disease likelihood) on all-cause mortality among patients in the Heidelberg subcohort using Kaplan-Meier curves. To assess CAD severity, stenosis percentages were derived from each coronary artery segment and were fitted to an unsupervised clustering analysis. Subsequently, CAD probability and cluster designations were integrated to examine disease severity.
Results: In our a-priori research, we identified 634 original abstracts, detailing 166, 181, 56, and 182 distinct miRNAs associated with ACS, CAD, DCM, and HFrEF, respectively. In the differential expression analysis, the univariate AUCs for the evaluated miRNAs indicated moderate diagnostic efficacy. ML models, particularly the XG-Boost algorithm, showed good diagnostic performance with class specific AUCs ranging from 0.86-0.94 and accuracy metrics spanning 0.82 to 0.92 when using the top selected miRNA features (m=50, Figure 1, Panel A). When patients were stratified into tertiles of predicted disease probability, the KM curves clearly showed a separation of all-cause mortality (Figure 1, Panel B). Finally, the predicted CAD probability was also clearly associated with a more severe CAD phenotype (p=0.005).
Discussion: We have validated several miRNAs that show altered expression levels in CVD and discriminate cases from controls, both as single markers or when combined in a multivariate signature. Furthermore, our findings indicate that the miRNA-predicted disease probability is associated with patient outcomes and disease severity. Thus, molecular biomarkers may serve as objective tools to assess the development and progression of CVD.
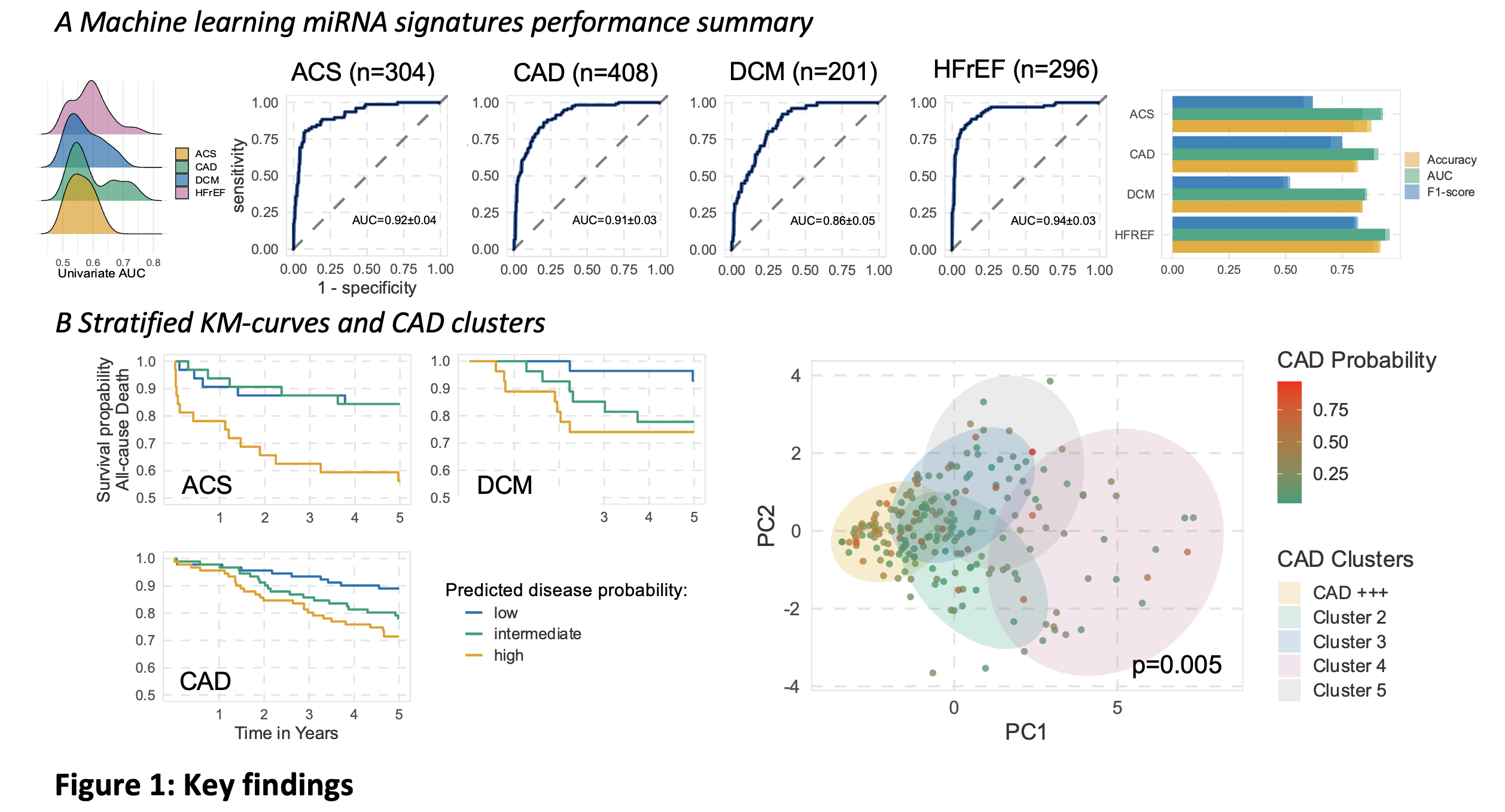